How AI-Powered Predictive Analytics Is Revolutionizing Case Outcomes
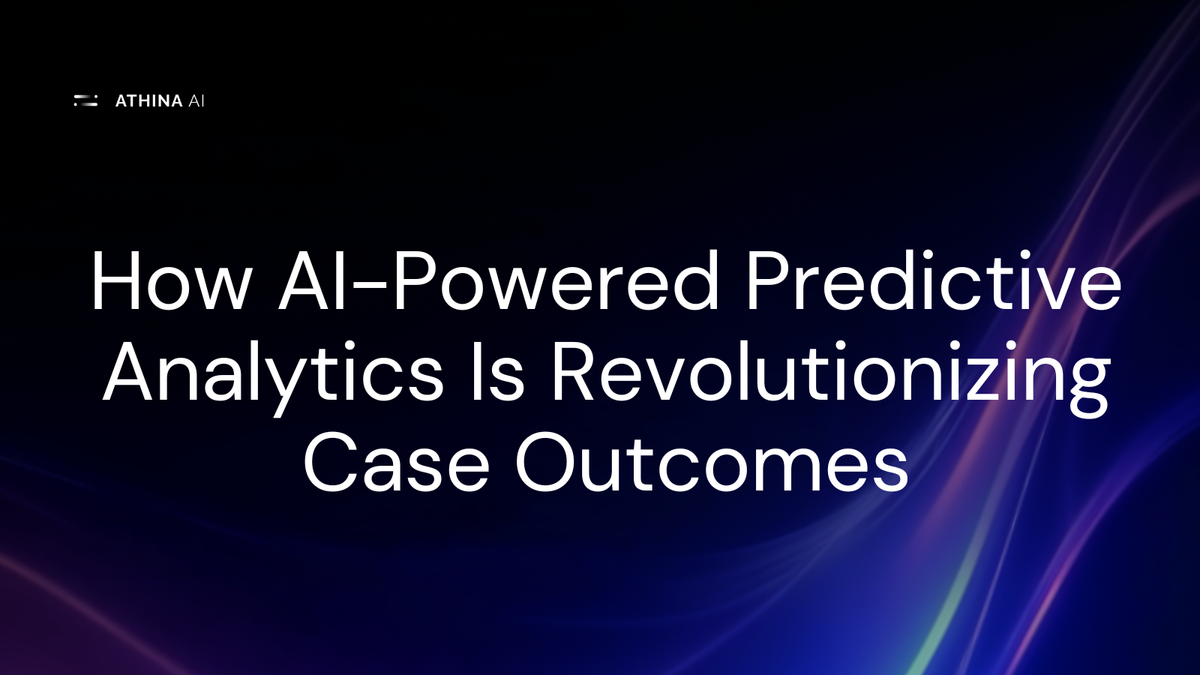
The legal and judicial landscape has long relied on historical data, expert opinions, and case precedents to determine case outcomes. However, with the advent of Artificial Intelligence (AI)-powered predictive analytics, the approach to legal decision-making is undergoing a seismic shift.
Predictive analytics leverages machine learning (ML) models, natural language processing (NLP), and Generative AI to forecast case outcomes with high accuracy. Additionally, generative AI is enhancing this capability by automating legal document generation, research, and analysis, making legal processes more efficient and data-driven.
In this blog, we will explore the role of AI-driven predictive analytics and generative AI in legal case outcomes, the underlying technologies, and the technical considerations that ensure accuracy and reliability.
The Role of AI in Predictive Analytics for Legal Cases
AI-powered predictive analytics plays a crucial role in multiple aspects of legal case management, including:
- Case Outcome Prediction: AI models analyze historical case data to forecast probable rulings.
- Sentencing Recommendations: Judges and attorneys use AI-generated insights to determine fair sentencing.
- Fraud Detection: AI identifies patterns of fraudulent activities in civil and criminal cases.
- Litigation Strategy Development: Lawyers leverage AI insights to tailor their case strategies based on probable outcomes.
- Automated Document Drafting: Generative AI models assist in drafting contracts, pleadings, and legal opinions with high precision.
These applications demonstrate how predictive analytics and generative AI are shifting legal decision-making from intuition-based approaches to data-driven strategies.
How AI-Powered Predictive Analytics and Generative AI Work
AI-powered predictive analytics and generative AI are transforming decision-making by leveraging data-driven insights and advanced machine learning models to forecast outcomes, automate legal processes, and enhance interpretability in complex scenarios. Lets go through it:
1. Data Collection and Preprocessing
Predictive analytics and generative AI models require vast datasets, including:
- Court judgments and case records
- Legal statutes and precedents
- Witness testimonies and depositions
- Financial and forensic reports
- Sentencing trends
- Existing legal contracts and templates
Data preprocessing techniques such as text normalization, entity recognition, and sentiment analysis are applied to structure unstructured data sources. AI-driven tools use Named Entity Recognition (NER) and topic modeling to extract essential legal elements like parties involved, charges, and legal reasoning.
2. Knowledge Augmentation with Retrieval-Augmented Generation (RAG)
Generative AI goes beyond traditional predictive models by integrating Retrieval-Augmented Generation (RAG), a hybrid approach combining retrieval mechanisms with large language models (LLMs). In legal analytics:
- Retrieval Phase: RAG retrieves relevant legal precedents, statutes, and case law from massive databases in real time, ensuring the AI has access to the most current and contextually appropriate information.
- Generation Phase: Using models like GPT or LLaMA, Generative AI synthesizes this retrieved data to produce coherent legal arguments, draft contracts, or summarize complex case histories.
- Contextual Relevance: Unlike static ML models, RAG enables dynamic updates by pulling in new rulings or legislative changes, making it ideal for adapting to evolving legal landscapes.
- Applications: Lawyers can query the system with natural language (e.g., “What’s the likely outcome for a fraud case with these specifics?”), and Generative AI provides tailored responses grounded in real-world legal data, complete with citations.
This process enhances legal research efficiency, reduces human error, and ensures outputs are both data-driven and legally sound.
3. Generative AI Models and Fine-Tuning for Legal Expertise
Generative AI leverages advanced architectures to power its legal applications:
- Transformer-Based Models: Models like BERT, GPT, or specialized legal variants (e.g., Legal-BERT) are fine-tuned on domain-specific datasets, such as court transcripts and legal opinions, to understand nuanced legal language and reasoning.
- Task-Specific Fine-Tuning: Through supervised learning on labeled legal texts, these models excel at tasks like automated contract drafting, summarizing lengthy judgments, or generating litigation strategies based on case similarities.
- Chain-of-Thought Prompting: Generative AI can simulate judicial reasoning by breaking down complex legal questions into logical steps, providing transparent and defensible outputs (e.g., “Here’s why this precedent applies”).
- Scalability: Cloud-based training on vast legal corpora allows these models to handle millions of documents, identifying patterns humans might miss, such as subtle shifts in sentencing trends or judicial preferences.
By combining natural language understanding with legal expertise, Generative AI delivers precise, actionable insights for attorneys and judges alike.
4. Ensuring Reliability and Ethical Deployment
The power of Generative AI in legal settings hinges on its reliability and ethical application:
- Hallucination Mitigation: Techniques like grounding responses in retrieved data (via RAG) reduce the risk of fabricated outputs, ensuring generated documents or predictions align with verifiable legal facts.
- Bias Detection and Correction: Regular audits of training data and outputs identify potential biases (e.g., skewed sentencing recommendations), with fine-tuning adjustments to promote fairness across demographics.
- Explainability: Generative AI incorporates tools like attention visualization to highlight which parts of a precedent or statute influenced its output, making decisions transparent to legal professionals.
- Regulatory Alignment: Models are designed to comply with privacy laws (e.g., GDPR) and ethical AI guidelines, with safeguards like anonymization of sensitive case data and audit trails for generated content.
These measures ensure that Generative AI not only enhances efficiency but also upholds the integrity and trustworthiness demanded by the legal system.
Why use Predictive AI: Key Benefits
AI-powered predictive analytics and generative AI offer transformative benefits across various domains, particularly in legal and decision-making contexts. Key advantages include:
- Improved Case Outcome Prediction
AI models enhance accuracy in legal decision-making by identifying case similarities, judge rulings, and precedent applicability. - Enhanced Efficiency in Legal Research
AI powered search tools like Perplexity, Grok, ChatGPT streamline legal research, reducing the time lawyers spend sifting through documents. - Better Sentencing Fairness
Predictive models analyze past sentencing trends to recommend fair and unbiased punishments. - Cost Reduction for Legal Firms
Automating case assessments and legal research lowers operational costs and enhances client service. - Automated Legal Document Generation
Generative AI drafts contracts, legal briefs, and client letters with high efficiency, reducing workload for attorneys.
Challenges and Ethical Considerations
While AI-powered predictive analytics and generative AI offer transformative benefits, they also introduce several challenges and ethical considerations that must be carefully addressed.
1. Data Privacy and Confidentiality
Legal data is highly sensitive, and AI systems must comply with GDPR, HIPAA, and other regulatory standards to ensure confidentiality.
2. Algorithmic Bias and Fairness
Bias in AI models can lead to unfair legal outcomes. Ensuring diverse training datasets and continuous bias audits is critical.
3. Legal Interpretability and Transparency
AI decisions must be explainable to judges, lawyers, and defendants to maintain legal system credibility.
4. Integration with Traditional Legal Systems
Courts and legal firms must establish AI governance frameworks to integrate predictive analytics seamlessly with human decision-making.
The Future of AI-Powered Legal Analytics
AI-powered predictive analytics and generative AI are set to redefine the future of law with advancements in:
- Automated Contract Analysis: AI will review and draft contracts with increased precision.
- AI-Driven Mediation Tools: Dispute resolution will leverage AI to provide fair settlements without lengthy court trials.
- Personalized Legal Assistance: AI-powered chatbots and legal assistants will offer tailored legal advice to clients.
- Blockchain-Integrated Legal Systems: Smart contracts and decentralized AI-powered case management systems will enhance security and efficiency.
Conclusion
AI-powered predictive analytics and generative AI are revolutionizing legal case outcomes by enabling data-driven decision-making, improving efficiency, and ensuring fair judgments. As AI technologies continue to evolve, legal professionals must adapt to AI-driven tools while addressing ethical and regulatory challenges. With proper governance and continuous model refinement, AI will become an indispensable ally in the pursuit of justice.
The future of law is not about replacing human judgment with AI but about enhancing it with intelligent, data-backed insights. As AI-powered predictive analytics and generative AI become more sophisticated, they will play a pivotal role in ensuring a more efficient, transparent, and equitable legal system.