Beyond Preferences in AI Alignment
Original Paper: https://arxiv.org/abs/2408.16984
By: Tan Zhi-Xuan, Micah Carroll, Matija Franklin, Hal Ashton
Abstract:
The dominant practice of AI alignment assumes
(1) that preferences are an adequate representation of human values
(2) that human rationality can be understood in terms of maximizing the satisfaction of preferences
(3) that AI systems should be aligned with the preferences of one or more humans to ensure that they behave safely and in accordance with our values.
Whether implicitly followed or explicitly endorsed, these commitments constitute what we term a preferentist approach to AI alignment. In this paper, we characterize and challenge the preferentist approach, describing conceptual and technical alternatives that are ripe for further research.
We first survey the limits of rational choice theory as a descriptive model, explaining how preferences fail to capture the thick semantic content of human values, and how utility representations neglect the possible incommensurability of those values.
We then critique the normativity of expected utility theory (EUT) for humans and AI, drawing upon arguments showing how rational agents need not comply with EUT, while highlighting how EUT is silent on which preferences are normatively acceptable.
Finally, we argue that these limitations motivate a reframing of the targets of AI alignment: Instead of alignment with the preferences of a human user, developer, or humanity-writ-large, AI systems should be aligned with normative standards appropriate to their social roles, such as the role of a general-purpose assistant.
Furthermore, these standards should be negotiated and agreed upon by all relevant stakeholders.
On this alternative conception of alignment, a multiplicity of AI systems will be able to serve diverse ends, aligned with normative standards that promote mutual benefit and limit harm despite our plural and divergent values.
Summary Notes
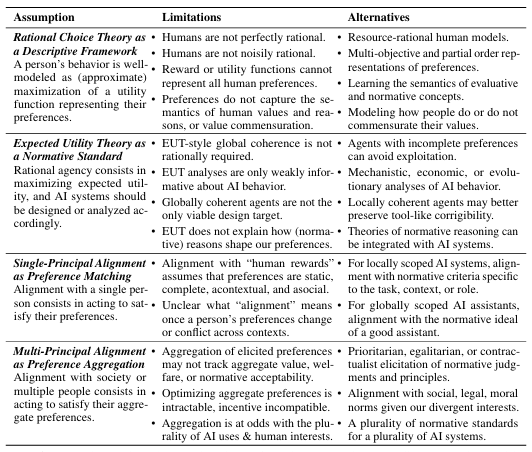
Table: Four theses that characterize the preferentist approach to AI alignment, along with a summary of their limitations and alternatives.
Introduction
As advancements in AI continue to accelerate, ensuring that AI systems align with human values becomes increasingly crucial.
Traditionally, AI alignment has relied heavily on the concept of human preferences, assuming that these preferences are static, rational, and adequately represent human values.
However, recent research by Tan Zhi-Xuan, Micah Carroll, Matija Franklin, and Hal Ashton challenges this preferentist approach, advocating for a more nuanced understanding of human values and their representation in AI systems.
The Preferentist Approach and Its Limitations
The preferentist approach in AI alignment is built on several key theses:
- Rational Choice Theory as a Descriptive Framework: Human behavior is modeled as the maximization of preference satisfaction, represented by utility functions.
- Expected Utility Theory as a Normative Standard: Rational agents should maximize expected utility, and AI systems should adhere to this standard.
- Single-Principal Alignment as Preference Matching: AI should maximize the preferences of a single human user.
- Multi-Principal Alignment as Preference Aggregation: AI should maximize the aggregated preferences of multiple users.
While these theses provide a structured framework, they fall short in several ways:
- Human Rationality: Humans are not perfectly rational; their decisions are influenced by cognitive limitations and biases.
- Complexity of Human Values: Utility functions often fail to capture the incommensurability and richness of human values.
- Preference Dynamics: Human preferences are dynamic and context-dependent, making static preference models inadequate.
- Social and Normative Influences: Preferences are shaped by social contexts and normative judgments, which are not always captured by traditional models.
Reframing AI Alignment: Beyond Preferences
The researchers propose a paradigm shift from aligning AI with static human preferences to aligning AI with the normative standards appropriate to their roles.
This approach recognizes the complexity and context-dependence of human values.
Key Methodologies and Innovations
To address the limitations of the preferentist approach, the researchers suggest several innovative methodologies:
- Resource-Rational Models: These models account for the bounded rationality of human decision-making, incorporating cognitive limitations and biases.
- Multi-Objective Representations: Instead of scalar utilities, AI systems can use vector-valued rewards or conditional preference networks to capture the plurality of human values.
- Contextual and Dynamic Preferences: AI systems should be designed to handle the dynamic and context-dependent nature of human preferences, aligning with the normative ideals relevant to specific tasks or roles.
Main Findings and Results
The study highlights several critical findings:
- Inadequacy of Scalar Rewards: Scalar reward functions are limited in expressing the complexity of human preferences, especially in contexts involving moral and practical dilemmas.
- Importance of Normative Standards: Aligning AI with normative standards rather than raw preferences can better capture the ethical and social dimensions of human values.
- Dynamic Preference Models: Preferences change over time due to learning, reflection, and social influences, requiring AI systems to adopt flexible and adaptive models.
Implications and Potential Applications
The implications of this research are profound for the future of AI alignment:
- Enhanced AI Safety: By aligning AI with normative standards, we can mitigate risks associated with preference manipulation and misalignment.
- Improved Human-AI Interaction: AI systems that understand and adapt to the dynamic nature of human preferences can provide more effective and ethical assistance.
- Broader Ethical Considerations: This approach encourages the integration of ethical reasoning and social norms into AI design, fostering more responsible AI development.
Conclusion
The shift from a preferentist approach to a normative standard-based alignment represents a significant advancement in the field of AI.
By recognizing the complexity and dynamism of human values, this research paves the way for more robust, ethical, and context-aware AI systems.
As AI continues to permeate various aspects of society, adopting these innovative methodologies will be crucial in ensuring that AI systems align with the diverse and evolving values of humanity.