Large Language Monkeys: Scaling Inference Compute with Repeated Sampling
Original Paper: https://arxiv.org/abs/2407.21787
By: Bradley Brown, Jordan Juravsky, Ryan Ehrlich, Ronald Clark, Quoc V. Le, Christopher Ré, Azalia Mirhoseini
Abstract:
Scaling the amount of compute used to train language models has dramatically improved their capabilities. However, when it comes to inference, we often limit the amount of compute to only one attempt per problem.
Here, we explore inference compute as another axis for scaling by increasing the number of generated samples.
Across multiple tasks and models, we observe that coverage - the fraction of problems solved by any attempt - scales with the number of samples over four orders of magnitude.
In domains like coding and formal proofs, where all answers can be automatically verified, these increases in coverage directly translate into improved performance.
When we apply repeated sampling to SWE-bench Lite, the fraction of issues solved with DeepSeek-V2-Coder-Instruct increases from 15.9% with one sample to 56% with 250 samples, outperforming the single-attempt state-of-the-art of 43% which uses more capable frontier models.
Moreover, using current API pricing, amplifying the cheaper DeepSeek model with five samples is more cost-effective and solves more issues than paying a premium for one sample from GPT-4o or Claude 3.5 Sonnet.
Interestingly, the relationship between coverage and the number of samples is often log-linear and can be modelled with an exponentiated power law, suggesting the existence of inference-time scaling laws.
Finally, we find that identifying correct samples out of many generations remains an important direction for future research in domains without automatic verifiers.
When solving math word problems from GSM8K and MATH, coverage with Llama-3 models grows to over 95% with 10,000 samples.
However, common methods to pick correct solutions from a sample collection, such as majority voting or reward models, plateau beyond several hundred samples and fail to fully scale with the sample budget.
Summary Notes
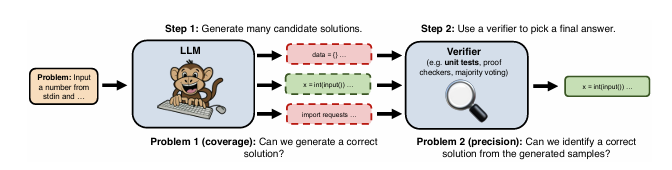
The repeated sampling procedure that we follow in this paper.
1) We generate many candidate solutions for a given problem by sampling from an LLM with a positive temperature.
2) We use a domain-specific verifier (ex. unit tests for code) to select a final answer from the generated samples.
Introduction
In recent years, the capabilities of large language models (LLMs) to solve complex tasks have seen remarkable improvements, driven primarily by scaling up model training.
However, an often overlooked dimension is scaling the computational resources used during inference.
This blog post explores the potential of repeated sampling as an effective method to enhance LLM performance.
The Research Question
The central question of this research is: Can we improve the performance of LLMs by scaling inference compute through repeated sampling?
The researchers investigate how generating multiple samples for a given problem can increase the likelihood of finding a correct solution.
Key Methodologies
The study employs a methodology known as repeated sampling. This involves generating numerous candidate solutions for a problem using an LLM and then using a verifier to select the best solution. The process is broken down into two main steps:
- Generate many candidate solutions: The LLM generates multiple solutions to a given problem using a positive sampling temperature to introduce variability.
- Use a verifier to pick a final answer: A domain-specific verifier, such as unit tests for code, is used to select the correct solution from the generated samples.
The effectiveness of repeated sampling is evaluated based on two key properties:
- Coverage: The fraction of problems for which at least one correct solution is generated.
- Precision: The ability to identify the correct solution from the generated samples.
Main Findings
Coverage Improvement
The research demonstrates that coverage improves significantly with an increased number of samples across various tasks and models.
For instance, when solving programming problems using the Gemma-2B model, coverage increased from 0.02% with one attempt to 7.1% with 10,000 attempts.
This improvement is observed to follow an approximate power law relationship between the logarithm of coverage and the number of samples.
Precision Challenges
While coverage improves with more samples, precision remains a challenge, especially in domains without automatic verifiers.
For example, in solving math word problems, common methods like majority voting or reward models plateau beyond several hundred samples and fail to fully scale with the sample budget.
Implications and Applications
Competitive Programming and Formal Proofs
In domains where solutions can be automatically verified, such as coding competitions and formal proofs, repeated sampling directly translates into improved task performance.
The study shows that models like Llama-3-8B-Instruct can outperform stronger models like GPT-4o when given multiple attempts.
Cost-Effectiveness
Repeated sampling also offers a cost-effective way to enhance model performance.
The research compares API pricing and finds that sampling five times from a cheaper model can solve more issues at a lower cost than using a single sample from a more expensive model like GPT-4o.
Real-World Applications
The ability to amplify weaker models through repeated sampling has significant real-world applications.
For example, in software engineering, this method can solve more issues in real-world GitHub repositories, as demonstrated on the SWE-bench Lite dataset.
Conclusion
Repeated sampling stands out as a promising method for scaling inference compute to improve LLM performance across various tasks.
While coverage sees substantial improvements, the challenge of precision highlights the need for better verification methods.
This research opens new avenues for optimizing both performance and cost in deploying LLMs.
Final Thoughts
This study underscores the potential of repeated sampling in enhancing the capabilities of LLMs. By balancing performance and cost, this method can be a game-changer in fields ranging from competitive programming to real-world software engineering.
Future research should focus on developing robust verifiers to fully harness the benefits of repeated sampling.