Top 10 AI Agent Papers from January 2025 shaping their future
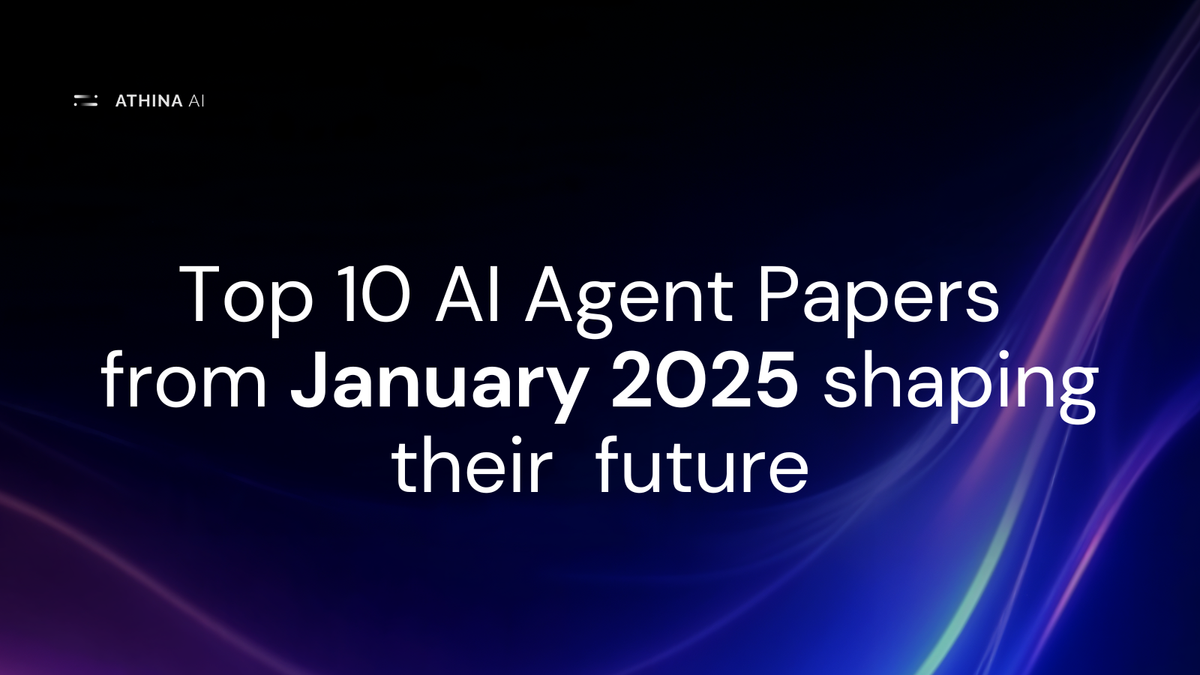
AI agents are getting smarter, faster, and more independent—and the research coming out now is laying the groundwork for what’s next.
We’ve selected 10 most relevant papers out of total 309 Agent papers released in January on Arxiv that tackle key challenges like governance, collaboration, reasoning, and automation. These papers introduce new frameworks, improve AI’s ability to interact with humans and systems, and explore better ways to ensure accountability and efficiency.
From enhancing AI-driven decision-making to integrating agents with Web3 and APIs, this research will shape how future AI agents operate. Lets dive in.
1) Beyond Browsing: API-Based Web Agents
AI agents traditionally interact with the web via browsing, but APIs offer a more efficient alternative. This study introduces API-Calling Agents and Hybrid Agents that combine web browsing with API access.
Experiments on the WebArena benchmark show that API-Based Agents outperform Browsing Agents, while Hybrid Agents achieve the highest success rate, improving performance by 24%.
Why it Matters:
Leveraging APIs alongside web browsing enhances AI agents' efficiency in online tasks. This approach could revolutionize automated web interactions, making AI-driven processes faster and more reliable.
2) Infrastructure for AI Agents
AI agents increasingly interact in open-ended environments, requiring new tools to manage both benefits and risks. Existing methods lack mechanisms to integrate AI agents with legal and economic systems.
The proposed agent infrastructure concept introduces technical systems and shared protocols to mediate agent interactions, ensuring accountability, shaping behaviors, and mitigating harm. Examples include authentication-based user-agent ties and regulatory mechanisms akin to HTTPS for the Internet.
Why it Matters:
Establishing agent infrastructure is crucial for safely integrating AI agents into society. It enables accountability, trust, and stability as AI systems become more autonomous and widespread.
3) Agentic Systems: A Guide to Transforming Industries with Vertical AI Agents
This paper explores the evolution of agentic systems in AI, focusing on Large Language Model (LLM) agents as the cognitive core. It proposes a standardization framework for Vertical AI agent design, introducing a Cognitive Skills module that enhances domain-specific inference.
The study outlines key components, operational patterns, and real-world applications of LLM-driven agents across industries.
Why it Matters:
Establishing standardized design patterns for Vertical AI agents ensures consistency, scalability, and adaptability, enabling more effective industry-specific AI solutions. This research advances AI’s role in transforming business and operational efficiencies.
4) DeepSeek-R1: Incentivizing Reasoning Capability in LLMs via Reinforcement Learning
The launch of DeepSeek-R1 shook the AI world, redefining the landscape of reasoning models.
By incorporating multi-stage training and cold-start data before reinforcement learning, it surpasses earlier limitations like poor readability and language mixing. DeepSeek-R1 achieves performance comparable to OpenAI-o1, demonstrating strong reasoning capabilities.
Why it Matters:
DeepSeek-R1 will be super helpful in shaping the future of AI agents, improving reasoning performance and accelerating innovation across AI applications.
5) IntellAgent: A Multi-Agent Framework for Evaluating Conversational AI Systems
Large Language Models (LLMs) are evolving into autonomous, task-oriented systems, but evaluating conversational AI remains challenging.
IntellAgent is a scalable, open-source framework that automates realistic, policy-driven benchmarking using graph modeling and interactive simulations. Unlike traditional static evaluations, it provides fine-grained diagnostics, identifies performance gaps, and supports modular integration for diverse domains and policies.
Why it Matters:
IntellAgent enhances the reliability and accountability of conversational AI by enabling more precise, scalable, and dynamic evaluations. Its open-source nature fosters collaboration and continuous improvement in AI development.
6) AI Agents for Computer Use: A Review of Instruction-based Computer Control, GUI Automation, and Operator Assistants
Instruction-based Computer Control Agents (CCAs) automate complex tasks on computers using natural language instructions.
This review formalizes the field, categorizing agents by environment, interaction methods, and learning approaches, emphasizing the shift from specialized agents to foundation models like LLMs and VLMs.
It evaluates 86 CCAs and 33 datasets, highlighting trends, challenges, and future directions for improving agent capabilities and deployment.
Why it Matters:
CCAs bridge human-computer interaction and automation, enabling more intuitive and accessible digital workflows. Understanding their development fosters advancements in AI-driven personal and professional productivity tools.
7) Governing AI Agents
AI is now shifting from generative models to autonomous agents capable of executing complex tasks independently. This transition raises governance challenges, which can be analyzed through principal-agent theory and agency law.
The paper identifies risks like information asymmetry and discretionary authority, critiques traditional solutions like monitoring and incentives, and proposes new legal and technical infrastructures to ensure inclusivity, visibility, and liability in AI governance.
Why it Matters:
Applying agency law to AI governance clarifies risks and solutions, helping build accountable and transparent AI systems. This approach ensures AI development aligns with legal and ethical standards.
8) Search-o1: Agentic Search-Enhanced Large Reasoning Models
Large reasoning models (LRMs) excel in stepwise reasoning but often struggle with knowledge insufficiency.
Search-o1 enhances LRMs by integrating an agentic retrieval-augmented generation (RAG) mechanism and a Reason-in-Documents module, allowing dynamic knowledge retrieval and refined document analysis. Experiments show significant improvements in complex reasoning tasks across various domains.
Why it Matters:
Search-o1 boosts the accuracy and reliability of AI reasoning by mitigating uncertainties. This advancement enhances AI's applicability in science, math, and coding, making intelligent systems more trustworthy and effective.
9) Multi-Agent Collaboration Mechanisms: A Survey of LLMs
LLM-based Multi-Agent Systems (MASs) enable AI agents to collaborate on complex tasks at scale, shifting from isolated models to coordination-driven solutions.
This survey explores MAS collaboration mechanisms, including actors, structures, and strategies, while presenting an extensible framework for future research. Applications span diverse fields like 5G/6G, Industry 5.0, and social systems, highlighting MASs' growing impact.
Why it Matters:
Advancing MASs fosters more intelligent, scalable, and cooperative AI solutions. This research paves the way for artificial collective intelligence, enhancing AI’s role in real-world problem-solving and automation.
10) Cocoa: Co-Planning and Co-Execution with AI Agents
Cocoa introduces interactive plans, a novel collaboration model for AI-assisted multi-step tasks in document editing. Users engage in Co-planning (joint action design) and Co-execution (shared task execution) to balance AI and human input.
Evaluations with researchers show that Cocoa enhances agent steerability while maintaining ease of use, outperforming traditional chat-based interactions.
Why it Matters:
Cocoa redefines human-AI collaboration by enabling structured, interactive workflows. This approach improves AI usability in complex tasks, fostering more effective and flexible user control.
Conclusion
The future of AI agents is being built right now, and these ten papers from arXiv offer a glimpse into what’s coming. From governance and collaboration to automation and reasoning, researchers are pushing the boundaries of what AI can do. As agents become more capable and independent, innovations like Web3 integration, multi-agent teamwork, and improved decision-making will play a crucial role in shaping their impact on the world.
Whether you’re an AI researcher, developer, or just curious about the future of intelligent systems, staying on top of this cutting-edge work is essential. The breakthroughs happening today will define how AI agents operate tomorrow—so keep watching this space!
Looking to streamline your AI development? Explore Athina AI — the ideal platform for building, testing, and monitoring AI features tailored to your needs.