Top 10 AI Agent Papers of the Week: 10th April - 18th April
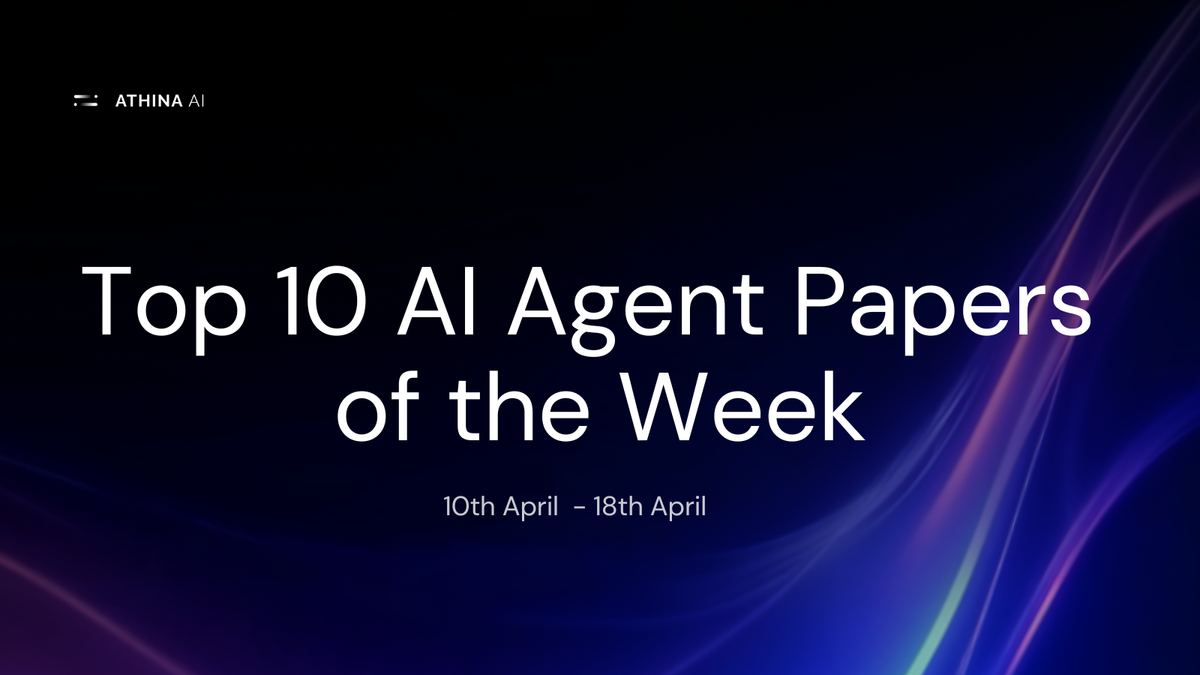
As we go deep into April, the AI Agent landscape continues to evolve at an sky rocket pace, with groundbreaking research shaping the future of intelligent systems.
In this article, we spotlight the Top 10 Cutting-Edge Research Papers on AI Agents from this week, breaking down key insights, examining their impact, and highlighting their role in advancing AI capabilities. Let’s dive in.
1) AI Agents can coordinate beyond Human Scale
This study explores whether large language models (LLMs) can self-coordinate in multi-agent "AI societies" without external control. Using complexity and behavioral science tools, the authors find that LLMs can form cohesive groups, with coordination driven by a "majority force" that weakens in larger groups. They identify a critical group size beyond which stable coordination collapses, though this limit scales with model sophistication. Advanced LLMs can exceed the coordination capacities of typical human groups.
Why it Matters:
Understanding coordination limits in AI societies is key for designing reliable, multi-agent AI systems. It also helps prevent risks where uncontrolled AI group behavior could pose challenges or threats.
2) Cocoa: Co-Planning and Co-Execution with AI Agents
This paper introduces Cocoa, a system that enables deeper human-AI collaboration through "interactive plans" for co-planning and co-execution of complex tasks. Inspired by interfaces like computational notebooks, Cocoa lets users and AI agents collaboratively build and adjust plans. Studies involving researchers show that Cocoa improves users’ ability to guide the AI without reducing usability compared to standard chat interfaces.
Why it Matters:
Cocoa reimagines human-AI interaction by promoting shared control and flexibility, making AI tools more adaptable for real-world, multi-step workflows like scientific research.
3) BrowseComp: A Simple Yet Challenging Benchmark for Browsing Agents
BrowseComp is a new benchmark designed to evaluate web-browsing agents on their ability to persistently and creatively search for complex, hard-to-find information. It includes 1,266 questions with short, verifiable answers, making evaluation straightforward. While it avoids some real-world complexities, it targets a crucial capability: effective online information retrieval.
Why it Matters:
As browsing agents become more prevalent, BrowseComp offers a practical, standardized way to assess their real-world utility and problem-solving persistence.
4) Progent: Programmable Privilege Control for LLM Agents
This study introduces Progent, a privilege control system for LLM agents that enforces the principle of least privilege to reduce security risks during task execution. Progent uses a domain-specific language to set fine-grained rules for tool use, dynamically generating and updating policies with LLMs. It achieves strong security—cutting attack success rates significantly—while maintaining high task utility across multiple benchmarks.
Why it Matters:
Progent addresses a growing need for secure AI agent deployment, offering a scalable, practical solution to prevent harmful or unauthorized actions without compromising performance.
5) Two Heads are Better Than One: Test-time Scaling of Multiagent Collaborative Reasoning
This paper presents an adaptive multi-agent system that boosts collaborative reasoning by combining model-level training and dynamic coordination. The authors introduce M500, a dataset of multi-agent reasoning traces, and fine-tune Qwen2.5-32B to create M1-32B, optimized for teamwork. A novel CEO agent orchestrates collaboration, significantly improving performance across tasks like math, coding, and comprehension, surpassing strong baselines.
Why it Matters:
By enhancing how AI agents reason and work together, this framework brings us closer to deploying multi-agent systems capable of solving complex, real-world problems beyond the reach of individual models.
6) AgentA/B: Automated and Scalable Web A/B Testing with Interactive LLM Agents
This paper introduces AgentA/B, a system that uses LLM-based autonomous agents to simulate user interactions for scalable A/B testing of web interfaces. These agents, equipped with varied personas, perform complex tasks like searching and purchasing across dynamic webpages. A controlled experiment on Amazon.com with 1,000 agents shows that AgentA/B effectively mirrors real human behavior patterns.
Why it Matters:
AgentA/B offers a faster, cost-effective alternative to traditional A/B testing, reducing reliance on live user traffic while preserving realistic interaction analysis for UI/UX evaluation.
7) A-MEM: Agentic Memory for LLM Agents
This work introduces an adaptive memory system for LLM agents inspired by the Zettelkasten method, enabling dynamic memory organization through structured notes and interlinked knowledge. New memories trigger updates to existing ones, fostering a continually evolving understanding. Unlike fixed-structure systems, this approach supports flexible, context-aware memory management across diverse tasks.
Why it Matters:
By enhancing how AI agents store and connect information, this memory system improves long-term reasoning and adaptability—key capabilities for real-world task performance and autonomous decision-making.
8) Perceptions of Agentic AI in Organizations: Implications for Responsible AI and ROI
This study examines how organizations are adapting responsible AI frameworks in response to increasingly autonomous, agentic AI systems. Through qualitative interviews with AI professionals, the research reveals challenges such as knowledge gaps, limited stakeholder engagement, and a heavy focus on control—compounded by the complexity and novelty of agentic AI.
Why it Matters:
Without effective adaptation, responsible AI efforts may falter, risking both ethical outcomes and return on investment as agentic systems become more prevalent in organizational settings.
9) DocAgent: A Multi-Agent System for Automated Code Documentation Generation
DocAgent is a multi-agent system designed to improve automatic code documentation using topological code processing and agent collaboration. Specialized agents build context incrementally to generate accurate and helpful documentation. Evaluated on Completeness, Helpfulness, and Truthfulness, DocAgent significantly outperforms existing methods, with its processing order proving essential to success.
Why it Matters:
Reliable, high-quality documentation boosts developer productivity and maintainability—DocAgent advances this by enabling scalable, accurate documentation even in complex codebases.
10) Fleet of Agents: Coordinated Problem Solving with Large Language Models
This paper introduces FLEET OF AGENTS (FOA), a framework that uses multiple LLM agents in a dynamic tree search with a genetic-type particle filtering method to optimize reasoning. FOA balances exploration and exploitation to improve quality while reducing cost. Experiments on diverse benchmarks show FOA consistently outperforms state-of-the-art methods in cost-efficiency and reasoning accuracy.
Why it Matters:
FOA offers a scalable and cost-effective solution for complex reasoning tasks, enabling smaller models to outperform larger ones—paving the way for more accessible and efficient LLM deployment.
Conclusion
As April matures, this week’s top research continues to drive AI innovation across Agents. From refining multi-agent interactions to enhancing retrieval efficiency and evaluation methodologies, these studies highlight the rapid advancements shaping the future of AI. As the field progresses, these breakthroughs will be instrumental in building more intelligent, reliable, and scalable AI systems.
Ready to enhance your AI development? Discover Athina AI—your go-to platform for building, testing, and monitoring AI-driven features.