Top 10 RAG Papers from January 2025
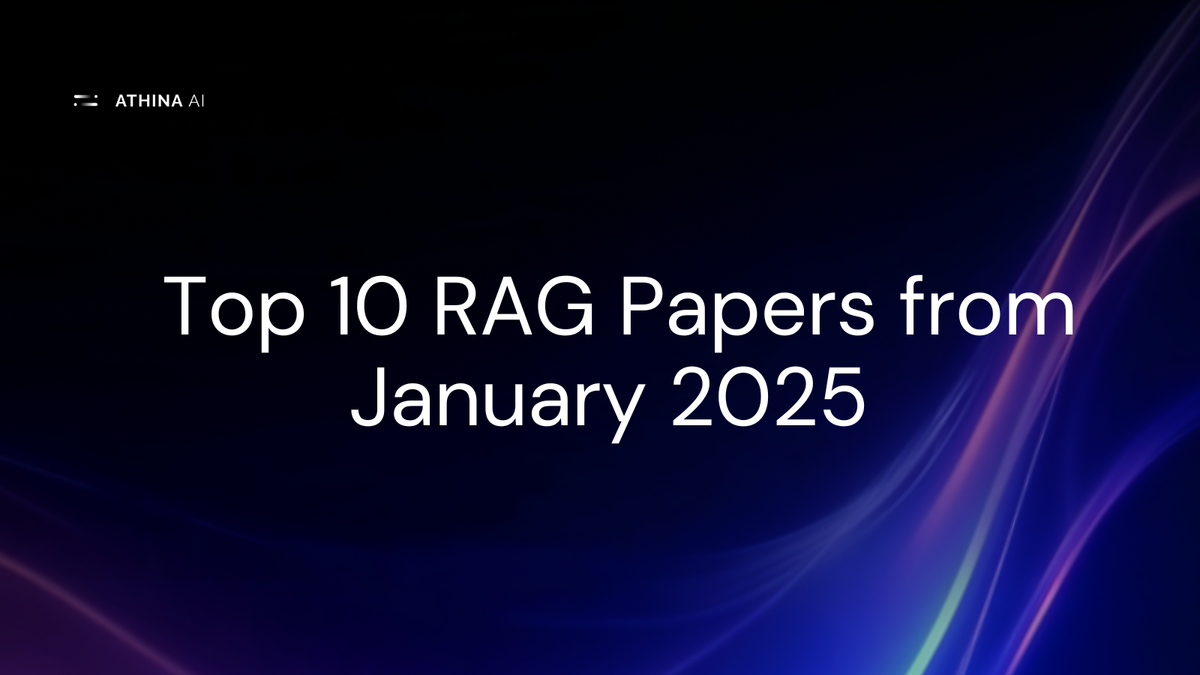
Retrieval-Augmented Generation (RAG) is evolving rapidly, becoming more efficient, accurate, and adaptable—and the latest research is setting the stage for its future advancements.
We’ve selected 10 of the most relevant papers from a total of 110 RAG papers released in January on Arxiv, that explore innovative RAG frameworks, improved retrieval strategies, security enhancements, and evaluation benchmarks. These works refine how AI integrates external knowledge, ensuring more reliable, context-aware, and scalable generation.
From optimizing retrieval mechanisms to refining knowledge grounding and response generation, this research will shape how future RAG models operate. Let’s dive in.
1) Retrieval-Augmented Generation with Graphs (GraphRAG)
This paper talks about GraphRAG, a novel extension of retrieval-augmented generation (RAG) that integrates graph-structured data to enhance knowledge retrieval and generation.
It defines a holistic framework with key components like query processing, retrieval, organization, and generation. The paper reviews domain-specific adaptations of GraphRAG and discusses associated challenges and future research opportunities.
Why it Matters:
GraphRAG unlocks the potential of graph-based knowledge for improving AI-driven reasoning and decision-making across various domains, making it a critical advancement in AI retrieval and generation techniques.
2) MiniRAG: Towards Extremely Simple Retrieval-Augmented Generation
MiniRAG is a novel, lightweight Retrieval-Augmented Generation (RAG) system designed for Small Language Models (SLMs) in resource-constrained environments.
It introduces a semantic-aware heterogeneous graph indexing mechanism and a topology-enhanced retrieval approach to improve efficiency without relying on complex language understanding. Experiments show that MiniRAG performs comparably to LLM-based methods while using only 25% of the storage.
Why it Matters:
MiniRAG enables efficient RAG deployment on limited-resource devices, expanding AI accessibility and practical applications in real-world, on-device scenarios.
3) VideoRAG: Retrieval-Augmented Generation over Video Corpus
VideoRAG is a novel Retrieval-Augmented Generation (RAG) framework that integrates video content for enhanced knowledge retrieval and response generation.
Unlike existing methods that rely primarily on text or convert videos into text descriptions, VideoRAG dynamically retrieves relevant videos and leverages both visual and textual information. It builds on Large Video Language Models (LVLMs) to process video content directly for improved retrieval and generation.
Why it Matters:
VideoRAG unlocks the rich multimodal potential of videos, improving AI’s ability to generate accurate, context-aware responses for applications in education, entertainment, and real-world decision-making.
4) SafeRAG: Benchmarking Security in Retrieval-Augmented Generation of Large Language Model
SafeRAG is a benchmark designed to evaluate the security vulnerabilities of Retrieval-Augmented Generation (RAG) systems against adversarial attacks.
It categorizes attacks into four types—silver noise, inter-context conflict, soft ad, and white Denial-of-Service—and introduces a manually curated dataset to test RAG robustness. Experiments on 14 RAG components reveal significant vulnerabilities, showing that even basic attacks can bypass current safeguards.
Why it Matters:
SafeRAG highlights critical security risks in RAG systems, urging the development of stronger defenses to prevent misinformation, manipulation, and service degradation in AI-driven applications.
5) Agentic Retrieval-Augmented Generation: A Survey on Agentic RAG
Agentic Retrieval-Augmented Generation (Agentic RAG) enhances traditional RAG by integrating autonomous AI agents to improve retrieval strategies, contextual refinement, and workflow adaptability.
Using agentic design patterns like reflection, planning, and tool use, it enables flexible and scalable multi-step reasoning for complex tasks. This survey explores its principles, architectures, applications, and implementation challenges.
Why it Matters:
Agentic RAG advances AI’s ability to handle dynamic, real-time information retrieval and decision-making, making it a crucial innovation for industries requiring accurate and adaptable AI solutions.
6) TrustRAG: Enhancing Robustness and Trustworthiness in RAG
TrustRAG is a security-focused framework designed to protect Retrieval-Augmented Generation (RAG) systems from corpus poisoning attacks.
It employs a two-stage defense: (1) K-means clustering with cosine similarity and ROUGE metrics to detect suspicious content, and (2) a self-assessment mechanism to identify and resolve inconsistencies between internal and external knowledge.
TrustRAG is a training-free, plug-and-play solution that enhances retrieval accuracy and resilience against attacks.
Why it Matters:
TrustRAG strengthens the security of AI-driven knowledge retrieval, ensuring reliable and trustworthy outputs in applications where misinformation can have serious consequences.
7) Enhancing Retrieval-Augmented Generation: A Study of Best Practices
This study explores key design factors influencing Retrieval-Augmented Generation (RAG) systems, including query expansion, retrieval strategies, and Contrastive In-Context Learning.
Through extensive experimentation, it analyzes the impact of language model size, prompt design, document chunking, and multilingual knowledge bases on response quality, offering actionable insights for optimizing RAG performance.
Why it Matters:
By systematically evaluating RAG configurations, this research enables the development of more efficient and adaptable AI retrieval systems, improving accuracy and relevance across diverse real-world applications.
8) Chain-of-Retrieval Augmented Generation
CoRAG (Chain-of-Retrieval Augmented Generation) enhances RAG by iteratively retrieving and reasoning over information before generating an answer, overcoming limitations of single-step retrieval.
Using rejection sampling, it generates intermediate retrieval chains for better query reformulation. Experiments show significant performance gains, particularly in multi-hop question answering, where it outperforms strong baselines by over 10 EM points and sets new state-of-the-art results on the KILT benchmark.
Why it Matters:
CoRAG improves factual accuracy and reasoning in complex queries, advancing AI’s ability to handle knowledge-intensive tasks with greater reliability and depth.
9) Fact, Fetch, and Reason: A Unified Evaluation of Retrieval-Augmented Generation
FRAMES is a high-quality dataset designed to evaluate LLMs' factuality, retrieval, and reasoning in end-to-end Retrieval-Augmented Generation (RAG) scenarios.
It introduces challenging multi-hop questions that require integrating information from multiple sources. Baseline results reveal that state-of-the-art LLMs struggle without retrieval, but a multi-step retrieval pipeline improves accuracy by over 50%, reaching 0.66.
Why it Matters:
FRAMES provides a unified benchmark for assessing and improving LLM-based RAG systems, ensuring more reliable, factually grounded AI responses in real-world applications.
10) LONG RAG: Evaluating Long-Context & Long-Form Retrieval-Augmented Generation with Key Point Recall
LONG2RAG is a new benchmark designed to evaluate Retrieval-Augmented Generation (RAG) systems on long-context retrieval and long-form response generation.
It includes 280 questions across 10 domains, with lengthy retrieved documents (avg. 2,444 words). The proposed Key Point Recall (KPR) metric assesses how well LLMs incorporate key points from retrieved documents into their responses.
Why it Matters:
LONG2RAG fills critical evaluation gaps, enabling the development of RAG systems that better handle extensive context and generate more informative, well-grounded responses.
Conclusion
The future of Retrieval-Augmented Generation (RAG) is evolving rapidly, and these recent advancements highlight the ongoing innovations in retrieval strategies, security, evaluation, and efficiency. From agent-driven adaptability to enhanced long-context retrieval and robustness against adversarial attacks, researchers are continuously refining how AI integrates and utilizes external knowledge.
Whether you're an AI researcher, developer, or enthusiast, staying informed about these breakthroughs is crucial. The improvements made today will shape the next generation of AI systems—making them more accurate, secure, and capable than ever before. Keep an eye on this space for what’s next in RAG!
Looking to streamline your AI development? Explore Athina AI — the ideal platform for building, testing, and monitoring AI features tailored to your needs.