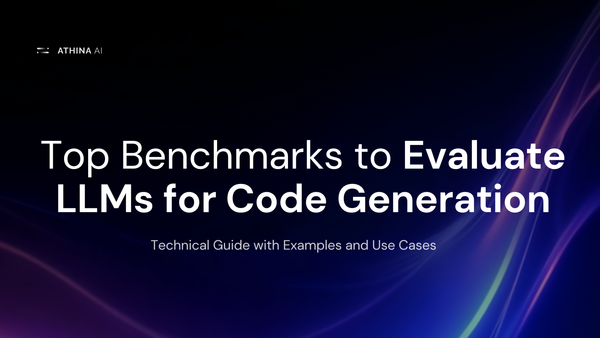
Top Benchmarks to Evaluate LLMs for Code Generation
From creating code snippets to debugging and program optimisation, Large Language Models (LLMs) have made amazing progress helping with software development. However, to evaluate their practical value, assessing these models calls for thorough benchmarking. Several benchmarks have been created to evaluate an LLM's capacity to manage programming assignments,